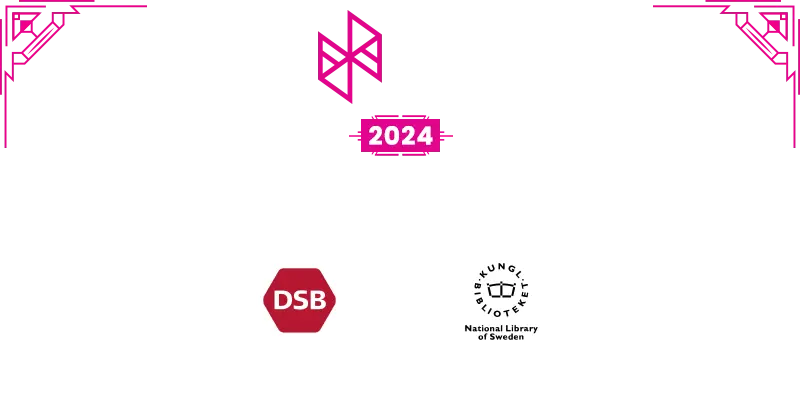
This award recognizes a public sector organization that has excelled in applying AI to enhance public services and deliver substantial value to society. The award celebrates innovative uses of AI that have resulted in improved service delivery, operational efficiency, and positive societal impact. The winning organization should demonstrate how AI has been effectively utilized to drive excellence in public administration, benefiting citizens and the community at large.
DSB is Denmark’s leading passenger rail operator, with over 140 years of history and nearly 500,000 daily travelers. Central to Denmark’s transport infrastructure, DSB is committed to providing reliable, safe, and sustainable transportation, guided by its motto: “Room for everyone on the journey towards sustainability.”
Financial Performance: In 2023, DSB achieved a pre-tax profit of DKK 425 million, significantly up from DKK 229 million in 2022. Passenger revenue increased by 8%, reflecting the growing demand for DSB’s services.
Customer and Travel Figures: In 2023, DSB recorded 162 million journeys, a 9% increase from 2022, driven by popular Orange tickets. S-trains achieved a record punctuality rate of 96%, while long-distance and regional trains faced some challenges.
Sustainability Efforts: DSB reduced CO2e emissions by 14% in 2023 and has set ambitious climate targets, aiming for a 30% reduction in supplier emissions by 2030 and net-zero by 2050. A green bond program has been established to support sustainable investments.
Future Outlook: DSB is modernizing its fleet with new electric IC5 train sets and Talgo carriages, with the first IC5 sets expected in 2027. The company is also working on implementing driverless S-trains for more efficient operations.
Conclusion: DSB’s focus on digital transformation, customer satisfaction, and sustainability makes it a key player in Denmark’s transportation future, continuously investing in innovative and environmentally friendly solutions.
At DSB, we are actively exploring innovation and the potential of AI technologies to enhance our operations and safety measures. Our dedicated team is utilizing computer vision to streamline incident reporting and improve safety by preventing unauthorized access on station tracks. We’re also implementing speech-to-text solutions to ease daily tasks for our operational colleagues. Additionally, we have a project focused on real-time delay prediction to provide better information to customers waiting for trains. This project involves building a model factory where ML models can be monitored, automatically retrained, and deployed into production as needed based on updated training data. We are also expanding our data architecture to support future projects and innovations.
DSB is a prime candidate for this award due to its innovative and impactful application of AI across multiple areas. By leveraging computer vision, DSB has enhanced safety on station platforms, preventing unauthorized access and improving overall operational efficiency. The implementation of speech-to-text technology has streamlined daily operations, making tasks easier for staff and ensuring smoother service delivery. Furthermore, DSB’s real-time delay prediction project, supported by an advanced model factory for continuous monitoring and retraining of ML models, has significantly improved the accuracy of customer information and overall passenger experience. These initiatives not only demonstrate DSB’s commitment to operational excellence but also highlight the substantial societal value provided through safer, more reliable, and efficient public transport services. DSB’s AI-driven innovations are a testament to its leadership, delivering tangible benefits to citizens and the community at large.
KBLab is a research and development laboratory at the National Library of Sweden. Its primary focus is on the AI-elevated accessibility to the library’s digital collections and on the creation of accessible, transparent, and robust language models. The lab engages in projects that leverage the library’s extensive collections of digital resources to develop tools and technologies for Swedish language processing. One of its central goals is to support both researchers and the public in accessing and analyzing large-scale text data in ways that are reliable and open.
KBLab is taking a two-pronged approach in training transformer models, tailoring each trajectory to meet the distinct needs of linguistic research and the Swedish language.
Trajectory 1: Predictive Models with Curated Data
The first trajectory focuses on creating predictive models that are trained on highly curated, meticulously assembled datasets drawn from the library’s vast collections. This approach emphasizes data precision and relevance, enabling models to be highly accurate and effective in predictive tasks. The data is selected and prepared with a high degree of scrutiny, ensuring that it is well-structured and relevant to specific predictive goals. Such rigorous curation allows these models to excel in tasks like text classification, document analysis, and other applications requiring robust, domain-specific predictions.
Trajectory 2: Fine-Tuning Multilingual LLMs for Swedish
The second trajectory is geared toward fine-tuning large multilingual models (LLMs) and foundational models, aiming to enhance their functionality and fluency in the Swedish language. By training these models with language-specific data and fine-tuning their parameters, KBLab aims to advance the capacity of these foundational models to understand, generate, and translate Swedish with greater precision and cultural nuance.
The first trajectory reflects a commitment to extracting refined insights from carefully chosen subsets of data, making it ideal for tasks that benefit from high fidelity and interpretability in Swedish. KBLab’s predictive models are released openly and have been downloaded more than four millions times from Huggingface.
The second trajectory leverages transfer learning and adaptation techniques, allowing multilingual models to achieve superior performance in Swedish-specific tasks without the need to build from scratch. Such advancements improve the models’ applications in natural language understanding, conversational AI, and document generation for Swedish, ultimately contributing to a more robust digital infrastructure for Swedish language processing.
Through these dual pathways, KBLab is not only advancing predictive capabilities rooted in Swedish literature and archival data but also enhancing multilingual models to better accommodate and serve Swedish language needs in diverse applications.